Image Deconvolution using Sparse Regularization
This numerical tour explores the use of sparsity regularization to perform image deconvolution.
Contents
Installing toolboxes and setting up the path.
You need to download the following files: signal toolbox and general toolbox.
You need to unzip these toolboxes in your working directory, so that you have toolbox_signal and toolbox_general in your directory.
For Scilab user: you must replace the Matlab comment '%' by its Scilab counterpart '//'.
Recommandation: You should create a text file named for instance numericaltour.sce (in Scilab) or numericaltour.m (in Matlab) to write all the Scilab/Matlab command you want to execute. Then, simply run exec('numericaltour.sce'); (in Scilab) or numericaltour; (in Matlab) to run the commands.
Execute this line only if you are using Matlab.
getd = @(p)path(p,path); % scilab users must *not* execute this
Then you can add the toolboxes to the path.
getd('toolbox_signal/'); getd('toolbox_general/');
Sparse Regularization
This tour consider measurements \(y=\Phi f_0 + w\) where \(\Phi\) is a convolution \( \Phi f = h \star f \) and \(w\) is an additive noise.
This tour is focussed on using sparsity to recover an image from the measurements \(y\). It consider a synthesis-based regularization, that compute a sparse set of coefficients \( (a_m^{\star})_m \) in a frame \(\Psi = (\psi_m)_m\) that solves \[a^{\star} \in \text{argmin}_a \: \frac{1}{2}\|y-\Phi \Psi a\|^2 + \lambda J(a)\]
where \(\lambda\) should be adapted to the noise level \(\|w\|\)
Here we used the notation \[\Psi a = \sum_m a_m \psi_m\] to indicate the reconstruction operator, and \(J(a)\) is the \(\ell^1\) sparsity prior \[J(a)=\sum_m \|a_m\|.\]
Image Blurring
Deconvolution corresponds to removing a blur from an image. We use here a Gaussian blur.
Parameters for the tour: width of the kernel (in pixel) and noise level.
setting = 1; switch setting case 1 % difficult s = 3; sigma = .02; case 2 % easy s = 1.2; sigma = .02; end
First we load the image to be processed.
n = 128*2; name = 'lena'; name = 'boat'; name = 'mri'; f0 = load_image(name); f0 = rescale(crop(f0,n));
Display it.
clf; imageplot(f0);
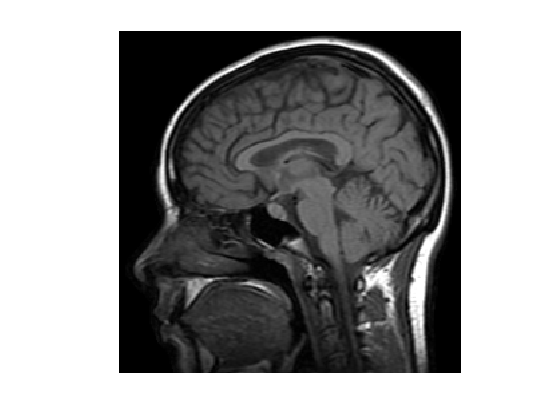
We build a convolution kernel. Since we are going to use Fourier to compute the convolution, we set the center of the kernel in the (1,1) pixel location.
Kernel.
x = [0:n/2-1, -n/2:-1]; [Y,X] = meshgrid(x,x); h = exp( (-X.^2-Y.^2)/(2*s^2) ); h = h/sum(h(:));
Useful for later : the Fourier transform (should be real because of symmetry).
hF = real(fft2(h));
Display the kernel \(h\) and its transform \(\hat h\). We use fftshift to center the filter for display.
clf; imageplot(fftshift(h), 'Filter', 1,2,1); imageplot(fftshift(hF), 'Fourier transform', 1,2,2);
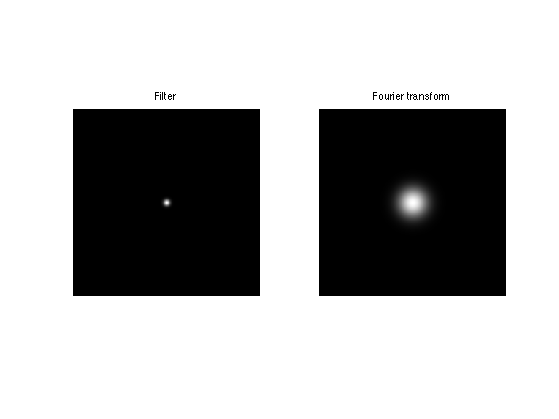
We use this short hand for the filtering. Scilab user should define a function in a separate file to perform this. Note that this is a symmetric operator.
Phi = @(x)real(ifft2(fft2(x).*hF));
Apply the filter.
y0 = Phi(f0);
Display the filtered observation.
clf; imageplot(f0, 'Image f0', 1,2,1); imageplot(y0, 'Observation without noise', 1,2,2);
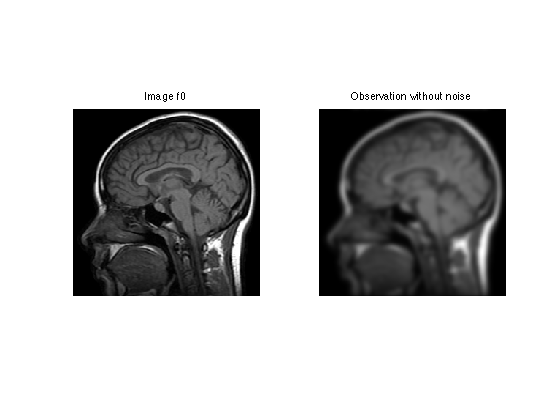
Add some noise of variance \(\sigma^2\), to obtain \(y=\Phi f_0 + w = f_0 \star h + w\).
y = y0 + randn(n,n)*sigma;
Display.
clf; imageplot(y0, 'Observation without noise', 1,2,1); imageplot(clamp(y), 'Observation with noise', 1,2,2);
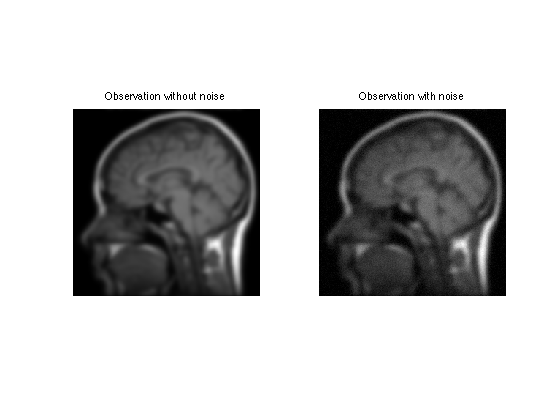
Soft Thresholding in a Basis
The soft thresholding operator is at the heart of \(\ell^1\) minimization schemes. It can be applied to coefficients \(a\), or to an image \(f\) in an ortho-basis.
The soft thresholding is a 1-D functional that shrinks the value of coefficients. \[ s_T(u)=\max(0,1-T/|u|)u \]
Define a shortcut for this soft thresholding 1-D functional.
SoftThresh = @(x,T)x.*max( 0, 1-T./max(abs(x),1e-10) );
Display a curve of the 1D soft thresholding.
clf;
T = linspace(-1,1,1000);
plot( T, SoftThresh(T,.5) );
axis('equal');
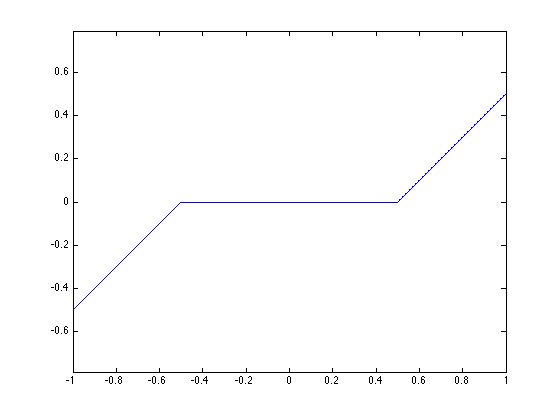
Note that the function SoftThresh can also be applied to vector (because of Matlab/Scilab vectorialized computation), which defines an operator on coefficients: \[ S_T(a) = ( s_T(a_m) )_m. \]
In the next section, we use an orthogonal wavelet basis \(\Psi\).
We set the parameters of the wavelet transform.
Jmax = log2(n)-1; Jmin = Jmax-3;
Shortcut for \(\Psi\) and \(\Psi^*\) in the orthogonal case.
options.ti = 0; % use orthogonality.
Psi = @(a)perform_wavelet_transf(a, Jmin, -1,options);
PsiS = @(f)perform_wavelet_transf(f, Jmin, +1,options);
The soft thresholding opterator in the basis \(\Psi\) is defined as \[S_T^\Psi(f) = \sum_m s_T( \langle f,\psi_m \rangle ) \psi_m \]
It thus corresponds to applying the transform \(\Psi^*\), thresholding the coefficients using \(S_T\) and then undoing the transform using \(\Psi\). \[ S_T^\Psi(f) = \Psi \circ S_T \circ \Psi^*\]
SoftThreshPsi = @(f,T)Psi(SoftThresh(PsiS(f),T));
This soft thresholding corresponds to a denoising operator.
clf; imageplot( clamp(SoftThreshPsi(f0,.1)) );
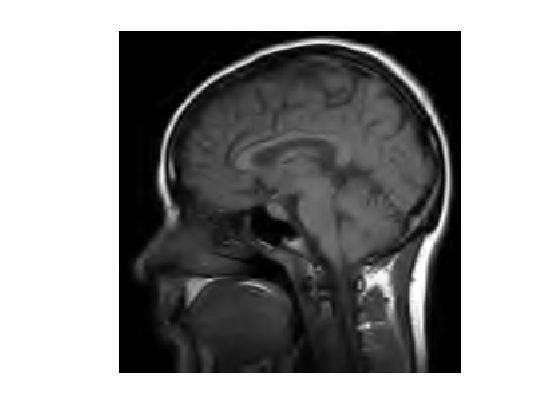
Deconvolution using Orthogonal Wavelet Sparsity
If \(\Psi\) is an orthogonal basis, a change of variable shows that the synthesis prior is also an analysis prior, that reads \[f^{\star} \in \text{argmin}_f \: E(f) = \frac{1}{2}\|y-\Phi f\|^2 + \lambda \sum_m \|\langle f,\psi_m \rangle\|. \]
To solve this non-smooth optimization problem, one can use forward-backward splitting, also known as iterative soft thresholding.
It computes a series of images \(f^{(\ell)}\) defined as \[ f^{(\ell+1)} = S_{\tau\lambda}^{\Psi}( f^{(\ell)} - \tau \Phi^{*} (\Phi f^{(\ell)} - y) ) \]
Set up the value of the threshold.
lambda = .02;
In our setting, since \(h\) is symmetric, one has \(\Phi^* f = \Phi f = f \star h\).
For \(f^{(\ell)}\) to converge to a solution of the problem, the gradient step size should be chosen as \[\tau < \frac{2}{\|\Phi^* \Phi\|}\]
Since the filtering is an operator of norm 1, this must be smaller than 2.
tau = 1.5;
Number of iterations.
niter = 100;
Initialize the solution.
fSpars = y;
First step: perform one step of gradient descent of the energy \( \|y-f\star h\|^2 \).
fSpars = fSpars + tau * Phi( y-Phi(fSpars) );
Second step: denoise the solution by thresholding.
fSpars = SoftThreshPsi( fSpars, lambda*tau );
Exercice 1: (check the solution) Perform the iterative soft thresholding. Monitor the decay of the energy \(E\) you are minimizing.
exo1;
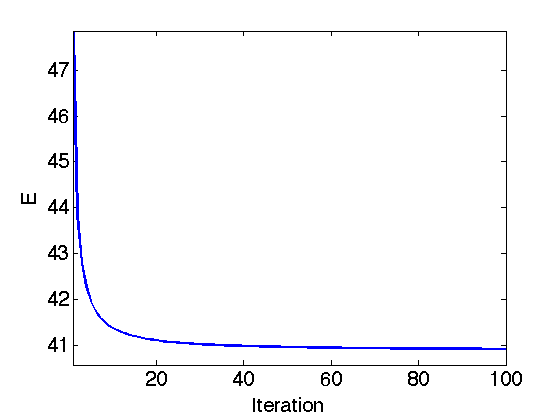
Display the result.
clf; imageplot(clamp(fSpars), ['Sparsity deconvolution, SNR=' num2str(snr(f0,fSpars),3) 'dB']);
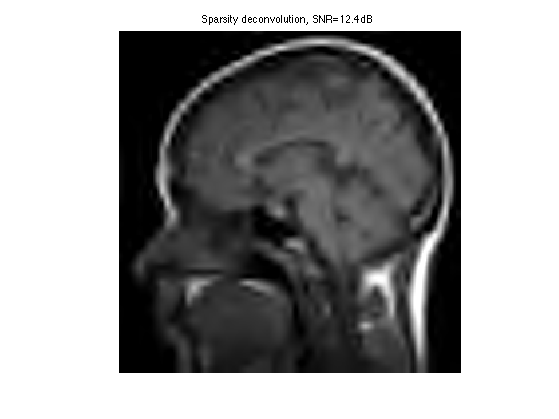
Exercice 2: (check the solution) Try to find the best threshold \(\lambda\). To this end, perform a lot of iterations, and progressively decay the threshold \(\lambda\) during the iterations. Record the best result in fBestOrtho.
exo2;
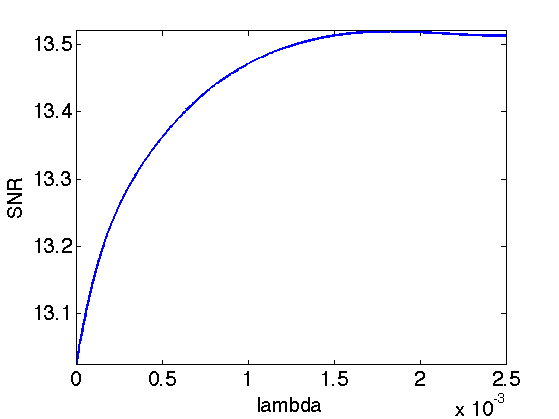
Display the result.
clf; imageplot(clamp(fBestOrtho), ['Sparsity deconvolution, SNR=' num2str(snr(f0,fBestOrtho),3) 'dB']);
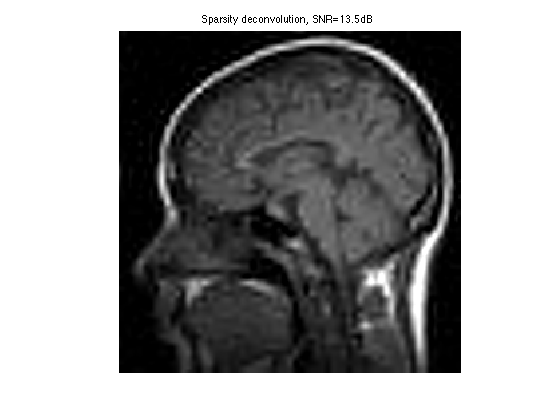
Deconvolution using Translation Invariant Wavelet Sparsity
Orthogonal sparsity performs a poor regularization because of the lack of translation invariance. This regularization is enhanced by considering \(\Psi\) as a redundant tight frame of translation invariant wavelets.
One thus looks for optimal coefficients \(a^\star\) that solves \[a^{\star} \in \text{argmin}_a \: \frac{1}{2}\|y-\Phi \Psi a\|^2 + \lambda J(a)\]
One should be careful that because of the redundancy of the wavelet tight frame, one should use a weighted \(\ell^1\) norm, where each coefficient is divided by the number of redundancy at each scale. \[J(a) = \sum_{j,k} u_j \|a_{j,k}\| \]
where the wavelet coefficients \(a_{m} = a_{j,k}\) are indexed by the scale (and orientation) \(j\) and the location \(k\).
Compute the scaling factor (inverse of the redundancy).
J = Jmax-Jmin+1; u = [4^(-J) 4.^(-floor(J+2/3:-1/3:1)) ];
Value of the regularization parameter.
lambda = .01;
Shortcut for the wavelet transform. Important: Note that PsiS is the shortcut for \(\Psi^*\), but Psi is the shortcut for \( \Xi = (\Psi^*)^+\) that satisfy \( \Xi \Phi^* f = f\).
options.ti = 1; % use translation invariance
Psi = @(a)perform_wavelet_transf(a, Jmin, -1,options);
PsiS = @(f)perform_wavelet_transf(f, Jmin, +1,options);
The forward-backward algorithm now compute a series of wavelet coefficients \(a^{(\ell)}\) computed as \[a^{(\ell+1)} = S_{\tau\lambda}( a^{(\ell)} + \Psi^*\Phi( y - \Phi\Xi a^{(\ell)} ) ). \]
The soft thresholding is defined as: \[\forall m, \quad S_T(a)_m = \max(0, 1-T/\|a_m\|)a_m. \]
The step size should satisfy: \[\tau < \frac{2}{\|\Phi\Psi\|^2}. \]
tau = 1.5;
Initialize the wavelet coefficients with those of the observations.
a = PsiS(y);
Gradient descent.
a = a + tau * PsiS( Phi( y-Phi(Psi(a)) ) );
Soft threshold.
a = SoftThresh( a, lambda*tau );
Important: keep in mind that the prior \(J(a)\) is a weighted \(\ell^1\) norm, it should thus be computed this way:
U = repmat( reshape(u,[1 1 length(u)]), [n n 1] ); Ja = sum(sum(sum( abs(a.*U) )));
Exercice 3: (check the solution) Perform the iterative soft thresholding. Monitor the decay of the energy.
exo3;
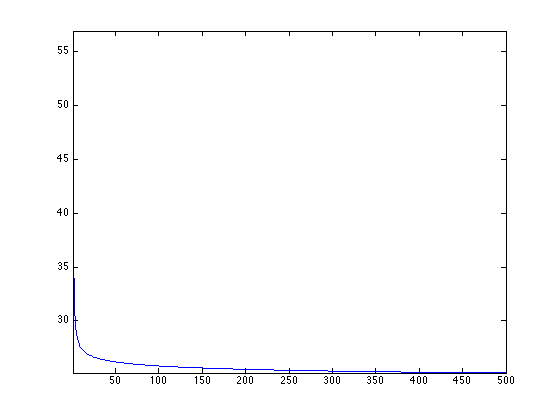
Perform the reconstruction.
fTI = Psi(a);
Display the result.
clf; imageplot(fTI);
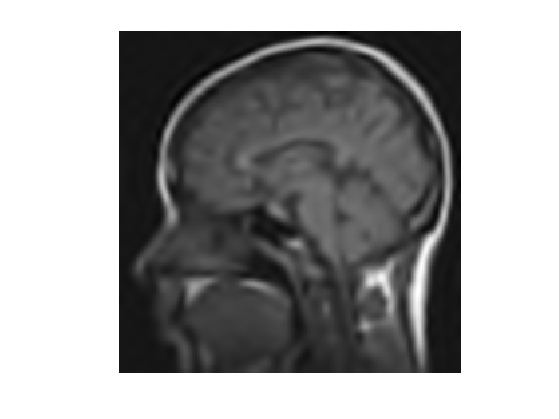
Exercice 4: (check the solution) Compute the optimal value of \(\lambda\), and record the optimal reconstruction fBestTI.
exo4;
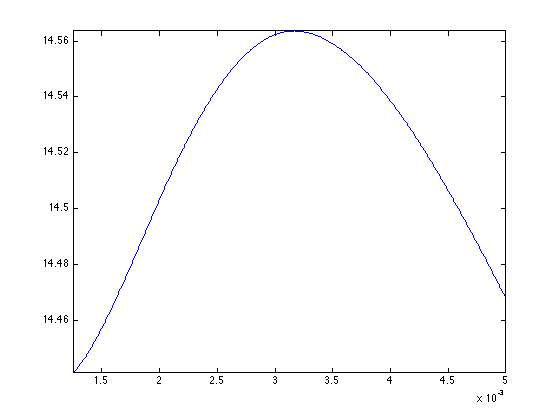
Display the result.
clf; imageplot(clamp(fBestTI), ['Sparsity deconvolution TI, SNR=' num2str(snr(f0,fBestTI),3) 'dB']);
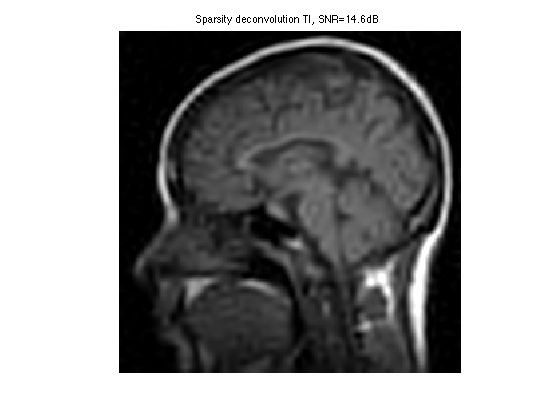
Exercice 5: (check the solution) Compare with the result of TV regularization, record the optimal TV result in fBestTV.
exo5;
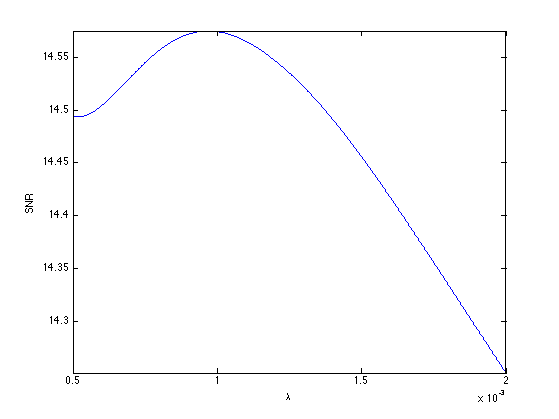
Display the result.
clf; imageplot(clamp(fBestTV), ['TV deconvolution, SNR=' num2str(snr(f0,fBestTV),3) 'dB']);
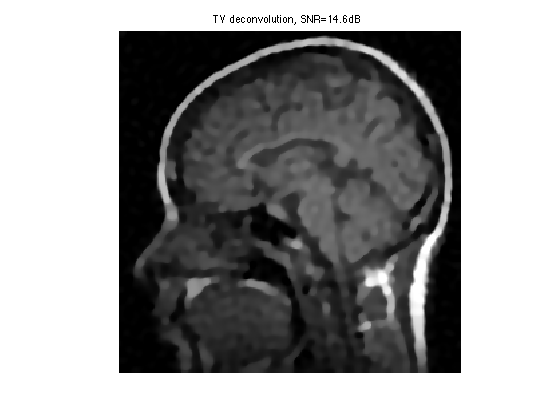